Thinking about taking a more structured approach to managing and governing the AI projects within your organization?
If you have this question on your mind, you’ve come to the right place.
Here at Shibumi, we’ve developed a recommended lifecycle approach for this very purpose. By using this AI lifecycle approach, you’ll help your organization effectively prioritize AI use cases, develop the most promising AI projects responsibly, make optimal use of resources and ensure that AI deployments yield meaningful outcomes for the business.
Stage 1: Problem Identification
The critical first step in an AI journey is to identify the business problems your organization wants to address with AI and the types of benefits most meaningful to you.
Start by identifying the top priorities of your organization. Is growing the top-line revenue by launching new product lines and expanding into new markets a top priority? Would you like to improve margins by improving operational efficiency and finding ways to avoid certain costs? Do you want to make significant improvements in customer satisfaction and retention by improving customer service? Or improve the competitive standing of your company by making your products more secure than anyone else’s? Do you need to meet your public commitments around sustainability?
You can leverage AI to help your enterprise accomplish any of these goals. The key is to figure out where you want to focus your AI initiatives in the near term vs. in the medium or long term.
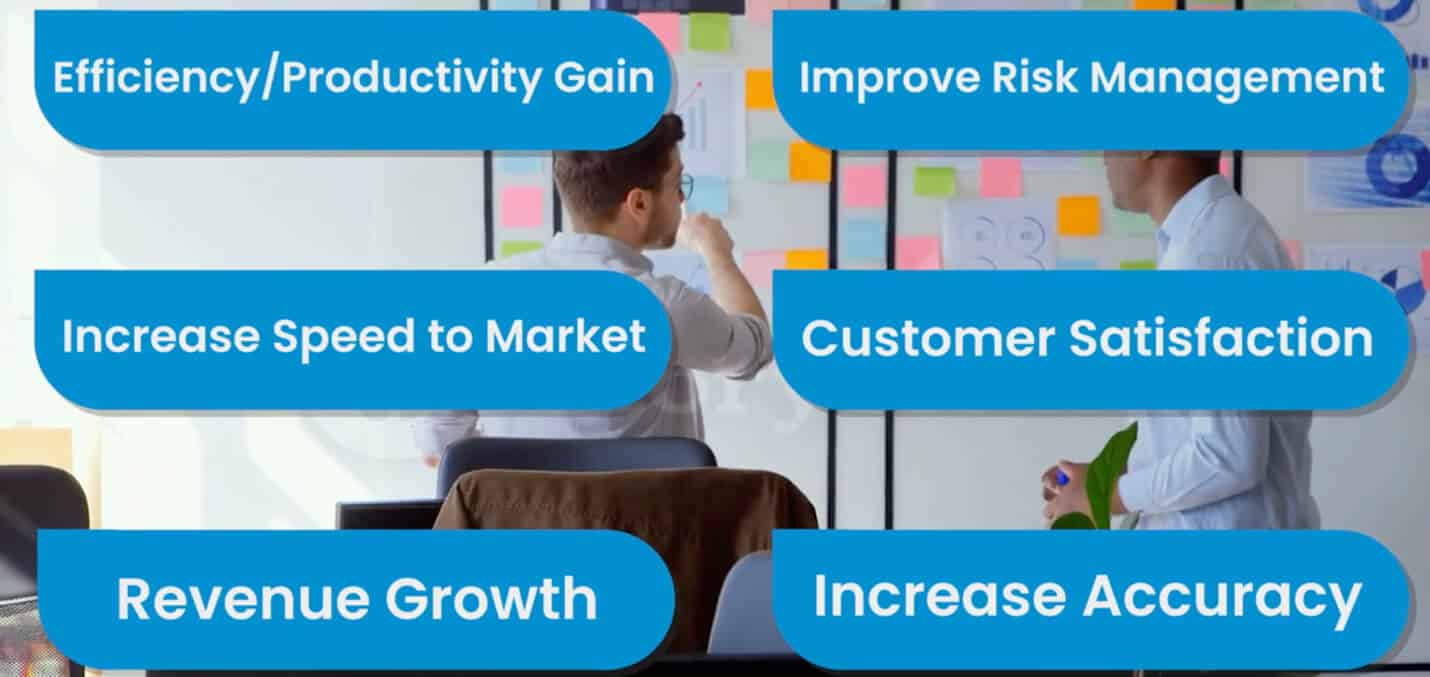
Common Business Drivers for Investing in AI
Identifying opportunities for AI
Start by examining your organization’s core mission and the function(s) and products your company relies on to generate revenue. How can AI help your organization improve its core product(s) and/or services? Or, should you change your product strategy because of AI?
For certain organizations, AI provides opportunities for significant innovation and can lead to the organization making major product strategy changes.
One company in this category is Intercom, a customer service solution that helps organizations automate and scale customer service. According to its Chief Product Officer, Paul Adams, Intercom went “all in” on AI in 2023, after he and other executives realized the power of large language models (LLMs). Intercom recognized that their target customers – customer support departments – are often answering support questions that can be handled by a LLM-powered bot trained on the organization’s existing help documentation.
Within a matter of months, Intercom built and released FIN, an AI-powered customer service bot that can converse naturally about support queries (FIN’s answers are trained on support content in its customers’ existing help center). After rolling out FIN to multiple customers, Intercom received feedback from its customers that more than half of their support inquiries are now answered by FIN.
Another example of a company directly impacted by advancements in AI is a cybersecurity software company. Cybersecurity software helps information security specialists be effective defenders. Yet, these professionals today are overwhelmed with threat data and it takes them too long to determine what’s a false alarm vs. a real threat. For the cybersecurity software firm, a natural place to apply AI is to incorporate algorithms in its software to help security professionals analyze their threat data faster and improve their threat detection and triage capabilities.
While it makes sense for these two companies to embed AI into their core product offerings, this may not be the right strategy for your organization. However, by taking the steps outlined below, you’ll find many other useful applications of AI.
Steps to take to discover business problems worth solving:
- Identify the business function and roles in your organization that are critical to how the organization makes revenue (e.g. software developers for software companies; consultants for management consultancies). What are the most time-consuming tasks performed by employees in these roles? Out of those, which ones can be sped up or eliminated by AI?
- Examine the core processes that drive how your organization generates revenue and see whether there’s room for AI to speed up or otherwise improve these processes. For example, the pharmaceuticals industry is heavily focused on using AI to speed up the lengthy new drug discovery process. Marketing and sales functions in enterprises are already making significant use of AI in their tech stack to improve the productivity and effectiveness of marketers and salespeople (though to date mostly through usage of tools for specific tasks, like getting AI summaries of sales calls from recording to using AI-embedded graphic design tools to speed up design creation).
- Talk to the leaders of each department. Ask them to list the most manual, time-intensive, repetitive processes or workflows in their department. Then, consider which processes can be sped up, enhanced or replaced with AI.
- Look at the customer journey customers go through for each of your product lines. Use your first-party data to identify the points along the customer journey where customers are dissatisfied or where there’s too much friction (e.g. customer waiting too long to get a response from your company).
- Identify the roles / job tasks in your organization where people routinely read, summarize and synthesize lots of text-based information (e.g. legal departments reviewing contracts). AI has gotten quite good at information summarization, synthesis and data anomaly detection.
- Identify decisions your organization makes on a regular basis that are costly and informed by lots of data (e.g. deciding which customer segments to target with paid media campaigns). Explore whether you can build or utilize AI models that make more accurate, cost-effective predictions than the status quo methods.
- Run a hackathon (also known as an innovation challenge). Give your employees time to learn and play around with pre-built large language models and open-sourced tools and see what ideas they come up with that can leverage these pre-built models or build on top of them.
When you start talking to people across your organization, you’ll likely find ample opportunities to deploy AI for the good of your employees, customers and your organization’s bottom line.
Given that every job function can benefit from AI, including a variety of employees in the ideation stage will help your organization uncover the widest set of high-value ideas.
Stage 2 – Initial Evaluation of AI Ideas
At this stage, the goal is to sort through the collection of AI project ideas to determine which ones deserve further consideration. To properly vet AI project ideas, decision-makers need to ask and answer the following questions for each AI opportunity/proposal:
- Is there a clearly defined business problem and a value hypothesis?
The individual or team that proposed the idea should outline a specific business problem and a value hypothesis for how AI can solve this problem. A good value hypothesis will describe how an AI use case will increase or decrease a specific business KPI and state the assumptions the value hypothesis is based on.
For example, a simple value hypothesis can look like this:
(AI Use Case “X”) will increase/decrease (Business KPI “Y”) by (“Z” %) amount
Teams should select and target KPIs closely aligned with organizational priorities and be sure to define these significant metrics early on. Unfortunately, many AI teams often leave the business KPI definition for much later in their projects. In the 2022 Gartner AI Use-Case ROI Survey, they found that mature AI organizations do the opposite–they’re much more likely to define their business metrics at the ideation phase of every AI use case than low-maturity ones.
KPIs don’t need to be financial–in fact, being overly focused on financial KPIs can lead organizations to overlook highly valuable use cases with strategic impact. Finding metrics that account for indirect business benefits such as improved accuracy (e.g. through eliminating room for human error), process cycle time, Net Promoter Score, and productivity are just as important and valuable.
- How much time and effort would be required to deploy this AI use case? Is it technically feasible? How complex is it from a technical standpoint?
- At a high level, are there major risks to implementing this idea? For instance, are there any obvious legal/ethical concerns to consider?
At this stage, the goal is not to be 100% accurate with the answers, but to guess based on information on hand. Remember, it’s always good practice to involve domain experts from product development, IT, Marketing, Operations and Finance and early in AI projects. Their experience and perspective are invaluable when generating AI value hypotheses and building strong business cases.
Stage 3 – Rank and Prioritize
This step happens right after you’ve culled your initial list. During this stage, you and others on your AI governance committee will do some deeper assessments and make some trade-offs. Your goal is to identify the projects that will make a sufficient impact and are feasible enough to try out. After the initial evaluation, you may notice your AI ideas can be grouped into one of two categories: 1) venture opportunity ideas or 2) business improvement ideas.
Venture opportunities are experimental in nature, dealing with AI techniques or capabilities the organization is less familiar with. In this case, we hypothesize a particular AI technique or capability may be what’s needed to solve a particular problem, but we don’t have enough expertise or experience to know whether this technique will work or not. Yet, the organization has the appetite to take some risks for the sake of learning and building expertise because it believes the reward may be significant.
Business improvement opportunities: If an organization has existing AI capabilities/knowledge and understands how to apply an AI capability to a business problem, these ideas can be considered “business improvement” ideas.
Unlike projects in the venture category, the organization has a firm idea how these AI use cases can benefit the business. Teams understand where and how to apply AI solutions and can use “hard” metrics such as time savings, cost savings, labor savings, cost avoidance, and revenue growth to measure the benefits of potential AI projects.
Generally, teams can implement project proposals in this category quicker than venture proposals with significantly higher odds of receiving a positive return.
Remember, when choosing an AI opportunity, it’s important to only compare opportunities in the same category, and not compare opportunities from different categories against one another.
Differences Between Venture Opportunities and Business Improvement Opportunities
Venture (Experimental) | Business improvement |
Leverages AI techniques or capabilities new to our organization | Leverages established AI techniques or capabilities we know how to apply |
Lower expectation of project paying off due to uncertainty of feasibility | Higher expectation of project paying off due to greater certainty of feasibility |
Lower expectation of project paying off because we don’t know if there’s AI-technique to business problem fit | Higher expectation of project paying off because we know there’s AI-technique to business problem fit. |
Longer time horizon to benefit realization | Shorter time horizon to benefit realization |
Higher tolerance for “failure” | Lower tolerance for “failure” |
Questions Ask to Prioritize AI Opportunities
Effective prioritization of AI projects should consider projects along three dimensions–Value, Feasibility, and Risk.
Value questions help identify the potential beneficial impact of a project. You may look at the potential project’s value to the organization, the value to customers, how scalable it would be to deploy the solution, whether it’s a foundation for unlocking innovation, and the expected time-to-value.
Risks should be evaluated for each potential AI project. What risks must you consider? Start with data–which will feed the all-important AI model training process. Will you have enough accurate, unbiased, usable (not in violation of any data privacy laws like HIPAA) data to train your AI models?
Your AI team must also consider the possible negative impact each AI deployment opportunity might have on specific population groups. Will this AI system potentially alienate certain employees or departments? If that potential exists, will the benefits outweigh the costs?
How about the risk of violating laws and regulations? You must think through which AI projects might need extra governance, and if so, is your organization prepared for that? Further, could cyber threat actors breach the AI system and compromise it?
Feasibility. How realistically doable is an idea? To deploy a new AI model, in addition to having sufficient data, you must also confirm the availability of other resources and tools. AI deployment success depends not only on having data scientists and Machine Learning (ML) engineers but also on knowledgeable business domain professionals who understand how to apply AI insights/recommendations to generate business value.
Further, don’t forget that change management is an aspect of feasibility. As you consider an idea, you must anticipate how the AI deployment could disrupt existing business processes, workflows and cause fear or concern for certain employee groups. What changes would deployment bring, and how can you effectively manage those changes for a seamless adoption?
Stage 4 – Prototype
In this stage, you and other decision-makers have chosen the list of promising ideas teams can start working on. These ideas are now for the prototype stage. We call this stage “Prototype” because in most cases, an AI project team will go through multiple rounds of a “train, test, refine” cycle to find/perfect the AI model deserving of wider deployment. Your AI project teams will go through each of the following steps multiple times:
- Build the AI model: Select a suitable algorithm for the type of problem you’re looking to solve. For instance, if you’re seeking to identify customers likely to be big spenders on your e-commerce site, you’d pick an algorithm suited for predicting outcomes such as a regression model.
- Augment data: Check to see if limitations exist in the dataset affecting the output of the initial model. If data limitations are found, augment the dataset to improve the model’s performance.
- Create a benchmark: Determine a suitable evaluation benchmark for judging your model’s performance. An effective benchmark is typically drawn from the experience of a human expert working with the same problem or from literature with the same algorithm or industry sector.
- Test and evaluate: Build multiple models to ensure the AI model demonstrates the expected “intelligence” and its performance meets or exceeds the benchmark.
- Explain the AI model: Document how the model works, the data used, and how the model parameters contribute to the expected AI outcome. “For your organization to embrace a new AI model that guides decision-making, the AI model must be understood by both business decision-makers and end-users who consume the output of AI models. That’s why it’s so important to document the value hypothesis for each round of testing, the success criteria, the actual results, and key learnings,” says Jingcong Zhao, Sr. Director of Product Marketing at Shibumi.
Stage 5– Implementation
The benefits of AI don’t just appear after an AI scientist has created a suitable, high-performing AI model worthy of deployment. All AI teams need a documented plan to advance a prototype into a solution operationalized in the business.
When designing your AI project implementation plan, remember to cover three tracks of work: 1) The technical work, 2) the risk mitigation work, and 3) user training/change management work.
According to De Silva & Alahakoon, authors of “An artificial intelligence life cycle: from conception to production“, the following technical and risk-related work should be done prior to implementing a new AI model:
- AI scientists need to hand over a prototypical and functional AI model to an AI/ML engineer, who checks if it’s computationally effective to be deployed for a broader audience.
- Security/privacy/UX experts need to assess the model focusing on risk assessment factors of privacy, cybersecurity, trust, usability, etc.
- AI models are integrated with existing system’s processes and sufficient testing is done to ensure the integration works reliably.
- AI/ML engineers do the AIOPs work necessary to develop the AI and data pipelines for operationalization.
- Depending on the industry sector and scope of the project, an expert panel, steering committee, or regulatory body may need to conduct a post-deployment technical and ethics review of the project.
Deploying an AI model or solution that replaces a human-driven process can be disruptive – so planning for change is a crucial aspect of deployment. Many activities influencing the ultimate success of an AI project (e.g., process re-engineering, team upskilling) are beyond the direct control of an AI team. Thus, it’s critical to bring in relevant business partners who are better suited to tasks necessary for driving user adoption and managing change.
Business readiness checklists can be a helpful tool as you work towards moving an AI solution to production.
To make this concrete, let’s say that we’re planning for the roll out of a new AI model that will tell our customer success team which customers are at high risk of churn and the expected value of retaining that customer. We’d want to verify the following information before the model is deployed:
- Do customer success managers understand why the business is deploying this AI churn risk prediction model?
- Are there any customer-facing workflows or processes that will need to change because of this deployment?
- Is the AI model’s output easy for customer success managers to understand? Do they know how to interpret the output, and do they know what to do based on it?
- Does the user interface/user experience of our customer portal need to change to allow customer success managers to consume the output(s) of the AI model?
- Does the customer success team need to be re-organized because of this change?
Stage 6: Benefit Realization
Monitoring and benefit realization may be last in sequence but not in importance. To develop AI systems responsibly, organizations need to have oversight of all AI models in production – continuously monitoring their performance and ensuring they’re functioning as intended. Without sufficient monitoring and maintenance, AI models will inevitably deteriorate and fail to produce the desired business value.
The performance of an AI model can drift over time, decreasing in accuracy due to the changing nature of the data. Model drift can be addressed by retraining the model with more recent data, capturing these changes or drifts. Models can also become stale due to changes in the problem or environment underlying the model design and addressing this requires rethinking model architecture, inputs, algorithm, and parameters.
Meanwhile, monitoring end-user activities helps gauge how models contribute to organizational functions. The method for gauging user adoption depends on use cases with metrics drawn from product telemetry, user questions, frequency of use, use/revision of documentation, feedback, and requests for features.
ROI and business benefits can be determined using metrics, such as reduced costs (due to reductions in turnaround time, human effort, human skills), increased revenue (due to new revenue workstreams, customer satisfaction, increased market share), and productivity gains (such as from reduced errors, low employee turnover, increased agility of teams, and improved workflows).
Although it may take some work–and support from other teams–to get ROI metrics, this data provides valuable evidence helping decision-makers feel comfortable investing more in the future.
Shibumi AI Value Accelerator: an AI Lifecycle Management Software
If you’re worried about how much time it would take to govern and manage all your AI projects consistently, the good news is that there are software solutions like Shibumi’s AI Value Accelerator that can help you do this at scale. With AI Value Accelerator, you can:
- Categorize AI projects by business goals and benefit types to drive tight alignment between projects and organizational priorities.
- Continuously gather AI ideas from your workforce and manage them centrally.
- Utilize business case and risk/feasibility assessment templates to document AI projects’ expected value, feasibility, risks, and costs and quickly understand the potential ROI of various projects.
- Manage each AI project through an iterative process and get the right people involved at the right time.
- Monitor the business benefits of deployed products and leverage automated reports to articulate the ROI of AI projects to executives.
You can learn more about AI Value Accelerator here.